Demystifying the Black Box: A Deep Dive into the World of Explainable AI (XAI)
Explore the critical realm of Explainable AI (XAI), where transparency meets technology. This comprehensive guide delves into the importance of understanding AI decision-making, the methodologies involved, and its impact on various industries and ethical AI practices.
Faheem Hassan
1/12/20242 min read
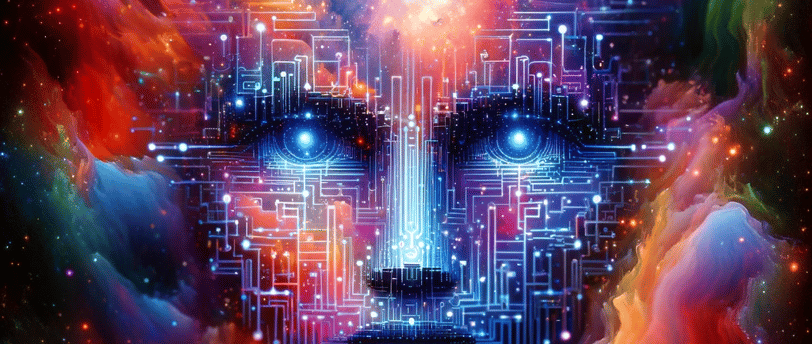
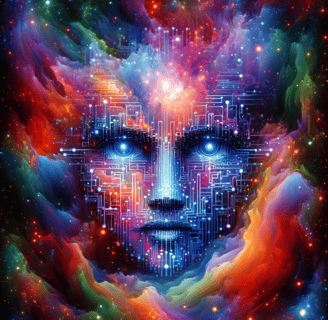
What is Explainable AI (XAI)? Explainable AI refers to techniques and methods that allow humans to comprehend and trust the results and outputs generated by machine learning algorithms. Unlike traditional AI systems, often deemed as 'black boxes' due to their opaque nature, XAI aims to bring clarity and understanding to the AI decision-making process.
Why is XAI Important?
Trust and Transparency: In sectors like healthcare and finance, where AI decisions have significant consequences, trust is paramount. XAI helps build this trust by making AI systems more transparent and understandable.
Compliance and Regulation: With increasing data privacy laws and regulations, XAI facilitates compliance by providing clear insights into how data is used and decisions are made.
Error Reduction: By understanding how AI models arrive at decisions, developers can identify and rectify potential errors more effectively.
Ethical AI Practices: XAI contributes to ethical AI development by ensuring that AI decisions are fair, unbiased, and ethically sound.
Methodologies in XAI: XAI incorporates various techniques to make AI systems explainable:
Data Visualization: Using graphical representations to illustrate how AI algorithms process and interpret data.
Model Interpretability Methods: Techniques like LIME (Local Interpretable Model-agnostic Explanations) and SHAP (SHapley Additive exPlanations) help in breaking down and explaining model predictions.
Interactive Tools: Tools that allow users to interact with AI models and understand their responses to different inputs and scenarios.
XAI in Action: From healthcare, where XAI can explain diagnostic decisions, to finance, where it elucidates credit scoring systems, the applications of XAI are vast and transformative. In AI-driven customer service, XAI helps in understanding and improving chatbot interactions, enhancing customer experience.
Challenges and Future Directions: While XAI is promising, it faces challenges like maintaining model performance while ensuring explainability and adapting explanations to different user groups. The future of XAI lies in advancing these techniques, making them more user-friendly, and integrating them into more complex AI systems.
Conclusion: Explainable AI is not just an add-on feature; it's a fundamental aspect of responsible AI development and deployment. As AI continues to evolve, XAI will play a crucial role in bridging the gap between advanced technology and human understanding, ensuring that AI systems are not only powerful but also transparent, accountable, and trustworthy.